Then, You cannot miss out Anakin AI!
Anakin AI is an all-in-one platform for all your workflow automation, create powerful AI App with an easy-to-use No Code App Builder, with Deepseek, OpenAI's o3-mini-high, Claude 3.7 Sonnet, FLUX, Minimax Video, Hunyuan...
Build Your Dream AI App within minutes, not weeks with Anakin AI!
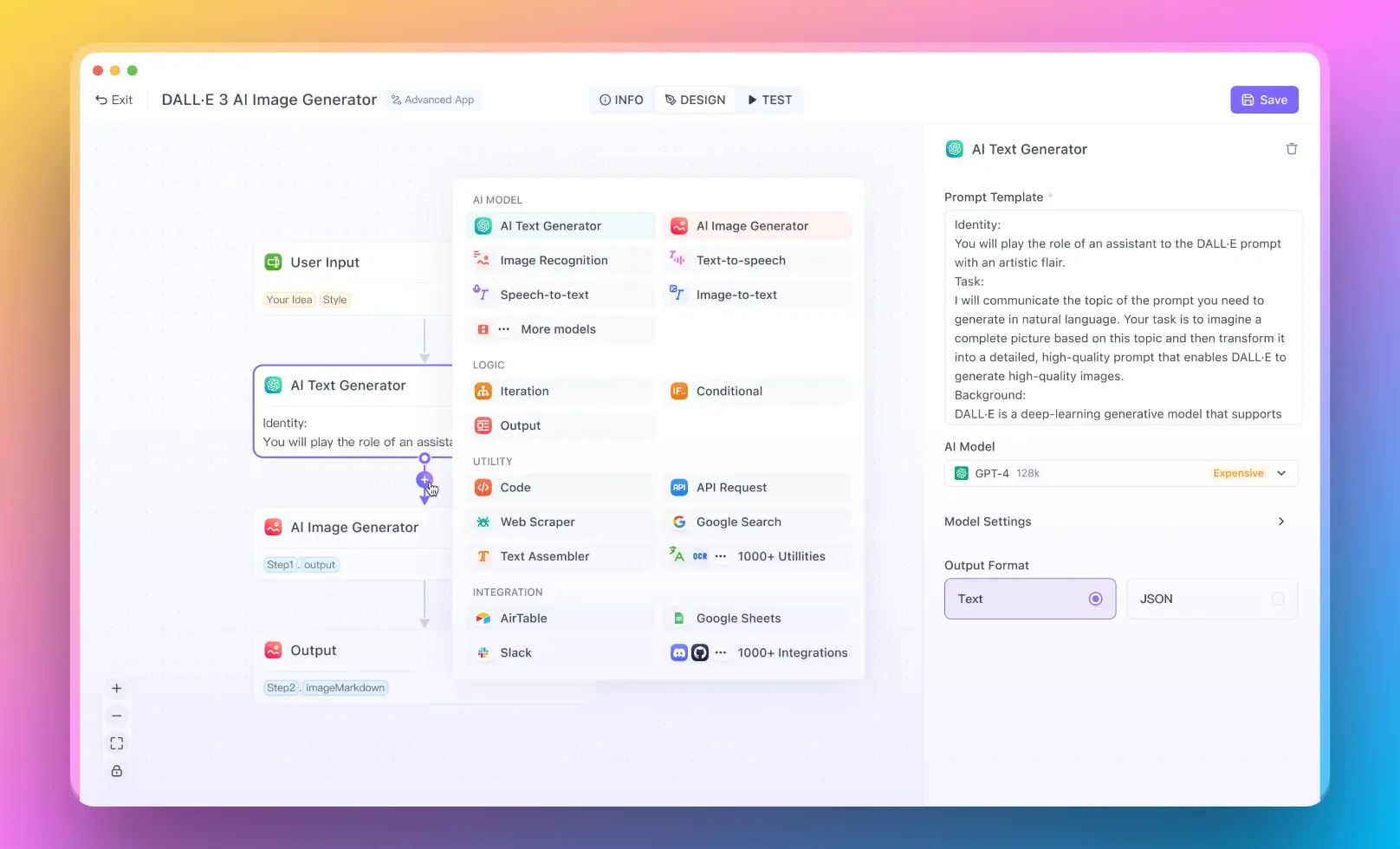
Artificial intelligence has made remarkable strides in understanding human communication, but accurately recognizing emotions across different modalities remains challenging. Alibaba's recently unveiled R1-Omni model represents a significant breakthrough in this domain, establishing itself as the industry's first application of Reinforcement Learning with Verifiable Reward (RLVR) to an Omni-multimodal large language model.
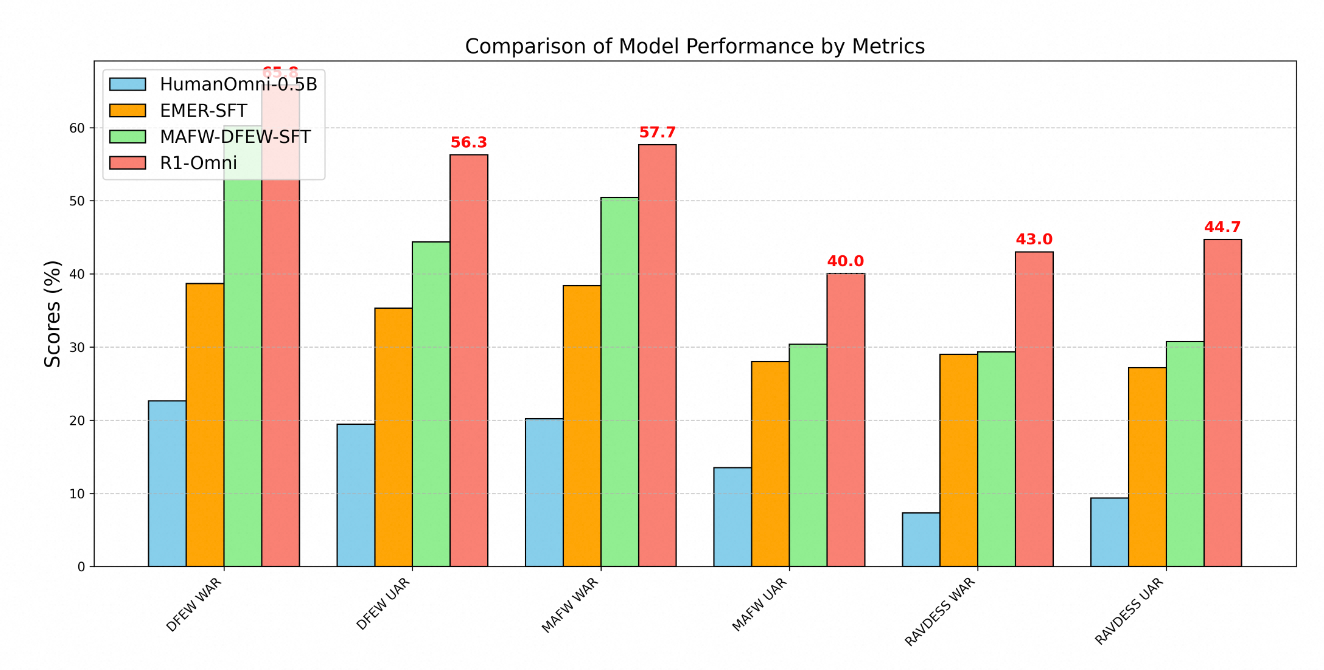
A New Approach to Emotion Recognition
Human emotions are complex and expressed through multiple channels simultaneously – facial expressions, vocal tones, body language, and verbal content. Traditional emotion recognition systems have struggled to integrate these diverse signals effectively, often failing to capture the nuanced interplay between visual and auditory cues that humans process instinctively.
R1-Omni addresses this challenge by leveraging a sophisticated reinforcement learning approach that enables the model to develop more refined understanding of how different modalities contribute to emotional states. Built upon the open-source HumanOmni-0.5B foundation, this innovative model demonstrates superior capabilities in reasoning, understanding, and generalization compared to conventionally trained systems.
"We focus on emotion recognition, a task where both visual and audio modalities play crucial roles, to validate the potential of combining RLVR with Omni model," note the researchers behind R1-Omni in their technical documentation.
Technical Architecture and Innovation
At its core, R1-Omni combines advanced multimodal processing with reinforcement learning techniques to create a more explainable and accurate emotion recognition system. The model processes visual inputs using the SigLIP-base-patch16-224 vision tower and handles audio through Whisper-large-v3, a powerful audio processing model capable of capturing subtle vocal cues that convey emotional information.
What distinguishes R1-Omni from previous approaches is its training methodology. While traditional supervised fine-tuning (SFT) trains models to predict emotion labels based on annotated examples, R1-Omni employs a reinforcement learning framework where the model is rewarded not just for correct predictions, but for demonstrating verifiable reasoning paths that lead to those predictions.
This novel approach promotes explainable connections between multimodal inputs and emotional outputs. Rather than simply labeling an emotion as "angry," R1-Omni can articulate specific visual cues (furrowed brows, tense facial muscles) and audio features (raised voice, rapid speech) that contribute to its assessment – a crucial capability for building trust in AI systems deployed in sensitive contexts.
Key Capabilities and Performance
R1-Omni demonstrates three key advancements over previous emotion recognition systems:
- Enhanced Reasoning Capability: The model provides detailed explanations for its classifications, connecting specific multimodal observations to emotional conclusions. This transparency represents a significant improvement over "black box" approaches that offer classifications without explanations.
- Improved Understanding Capability: Compared to models trained through supervised fine-tuning, R1-Omni demonstrates substantially better accuracy in emotion recognition tasks. This suggests the reinforcement learning approach helps develop more nuanced representations of emotional states that better align with human judgments.
- Stronger Generalization Capability: Perhaps most impressively, R1-Omni exhibits remarkable performance on out-of-distribution data – scenarios that differ from its training examples. This ability to generalize beyond specific training contexts is crucial for real-world applications.
The technical superiority of R1-Omni is clearly demonstrated through performance metrics across multiple emotion recognition benchmarks. Testing on three key datasets – DFEW, MAFW, and RAVDESS – provides comprehensive evaluation of the model's capabilities on both in-distribution and out-of-distribution data.
On the DFEW dataset, R1-Omni achieved a weighted average recall (WAR) of 65.83% and an unweighted average recall (UAR) of 56.27%, substantially outperforming both the baseline HumanOmni-0.5B model (22.64% WAR) and the MAFW-DFEW-SFT model (60.23% WAR) that was directly fine-tuned on the training sets.
Even more telling is the model's performance on out-of-distribution data. When tested on the RAVDESS dataset, which wasn't used during training, R1-Omni achieved 43% WAR and 44.69% UAR – dramatically better than the base model (7.33% WAR) and substantially higher than supervised fine-tuned alternatives (29.33% WAR).
Training Methodology
The development of R1-Omni followed a sophisticated two-stage training process:
First, in the "cold start" phase, researchers initialized the model using HumanOmni-0.5B and fine-tuned it on a carefully curated dataset consisting of 232 samples from the Explainable Multimodal Emotion Reasoning dataset and 348 samples from the HumanOmni dataset. This provided foundational capabilities while emphasizing explainable reasoning processes.
The second stage employed Reinforcement Learning with Verifiable Reward using a substantially larger dataset comprising 15,306 video samples from the MAFW and DFEW datasets. This reinforcement learning phase was critical in developing the model's advanced reasoning and generalization capabilities.
Throughout training, the process prioritized not just accurate classification but also the development of verifiable reasoning paths. Training examples typically included both emotion labels and structured thinking processes connecting observations to conclusions. This approach encouraged the model to develop explainable connections rather than simply learning statistical correlations.
Real-World Applications
The capabilities demonstrated by R1-Omni open numerous possibilities across various domains:
- Mental Health Support: The model could assist therapists by providing objective assessments of patients' emotional states, potentially identifying subtle emotional cues that might otherwise be missed.
- Education: Similar systems could help teachers gauge student engagement and emotional responses to learning materials, enabling more responsive educational approaches.
- Customer Service: R1-Omni's technology could enhance automated customer service systems by recognizing and appropriately responding to customer emotions, improving satisfaction rates.
- Content Analysis: The model could analyze emotional content in videos and audio recordings for market research, media analysis, and content moderation.
The model's explainability is particularly valuable in these contexts, as it allows human operators to understand and validate the reasoning behind AI-generated emotional assessments. This transparency builds trust and facilitates effective human-AI collaboration, essential for widespread adoption in sensitive domains.
Future Development
According to the project's roadmap, future developments for R1-Omni include integrating the source code of HumanOmni, releasing a more detailed reproduction process, open-sourcing all training data, developing inference capabilities for single-video and single-audio modality data, and releasing results from a larger 7B version of the model.
These planned enhancements will further increase the model's accessibility and utility for researchers and developers, potentially accelerating progress in the field of multimodal emotion recognition.
Conclusion
Alibaba's R1-Omni represents a significant advancement in AI-based emotion recognition through its innovative application of reinforcement learning techniques to multimodal understanding. By enhancing reasoning capabilities, improving accuracy, and demonstrating superior generalization to novel scenarios, R1-Omni pushes the boundaries of what's possible in emotional AI.
As we move toward more natural human-computer interaction, systems like R1-Omni that can accurately recognize and respond to human emotions across different communication channels will play an increasingly important role. The model's emphasis on explainability and generalization addresses critical limitations of previous approaches, setting a new standard for responsible and effective emotion recognition technology.
By combining the strengths of reinforcement learning with multimodal processing capabilities, Alibaba has created not just an improved emotion recognition system, but potentially a new paradigm for how AI systems can learn to understand the subtle complexities of human communication.