The AI community has been buzzing about Meta’s release of Llama 3.2, an innovative lineup of vision and text models designed for both edge devices and larger infrastructure setups. This new generation of models, including 1B, 3B, 11B, and 90B variants, brings advanced capabilities for on-device use, vision tasks, and large-scale inference. At Anakin AI, we proudly support Llama 3.2 alongside other top-tier models such as ChatGPT, Gemini, and Claude, offering users a seamless AI experience through our platform.
Let’s dive into the benchmarks and explore what makes Llama 3.2 a leader in open, customizable AI models for various use cases.
Key Takeaways: Llama 3.2’s Edge and Vision Power
- Lightweight Vision Models: Llama 3.2 includes 11B and 90B vision models, optimized for image understanding tasks such as captioning, document-level analysis, and visual reasoning. These models are perfect for on-device AI solutions, similar to the apps available on Anakin AI, such as the AI Clothes Remover or the Unfiltered AI Image Generator, which allow users to engage in powerful image-based tasks.
- Text-Only Models for Edge Use: The lightweight 1B and 3B models are optimized for on-device use, with a focus on tasks like summarization and rewriting. For example, apps like the Happy New Month Messages Generator and Goodnight Message Generator can benefit from these models to offer high-quality, personalized outputs.
- Cross-Platform Ecosystem: Llama 3.2 supports Qualcomm, MediaTek, and ARM processors, making it an efficient choice for mobile and edge applications. This is similar to how Anakin AI optimizes AI performance across multiple models, offering apps like the Deepfake AI Image Generator for users interested in seamless, cross-platform AI experiences.
Benchmarks: How Does Llama 3.2 Compare?

The benchmarks provided by Meta highlight how Llama 3.2 performs across various tasks, from vision to multilingual capabilities. Here are some highlights:
1. Lightweight Instruction-Tuned Benchmarks

The first table presents benchmarks across several categories, measuring performance in tasks related to reasoning, tool use, math, long context, and multilingual capabilities.
General NLP and Reasoning Benchmarks
- MMLU (5-shot): This benchmark evaluates a model’s performance in various domains such as humanities, STEM, and others. Llama 3.2 3B scores 63.4, outperforming Gemma 2B IT at 57.8 but trailing behind Phi-3.5-mini IT at 69.0.
- TLDR9: Measures summarization and comprehension abilities. Llama 3.2 1B scores 16.8, and the 3B version improves this score to 19.0, showing the model’s gains with increased parameters.
- IFEval: A comprehensive test of natural language understanding. Here, Llama 3.2 3B scores an impressive 77.4, beating Gemma 2B IT (61.9) and Phi-3.5-mini IT (59.2).
Tool Use Benchmarks
- BFCL V2: A test for how well the model uses external tools or APIs. Llama 3.2 3B performs much better than its 1B counterpart (67.0 vs. 25.7), which indicates a major leap in tool-use capabilities with higher model size.
- Nexus: Tests knowledge integration. Llama 3.2 3B outperforms Gemma 2B IT and Phi-3.5-mini IT by a large margin.
Mathematical and Reasoning Benchmarks
- GSM8K and MATH (5-shot): These tests evaluate the model’s ability to solve math problems. Llama 3.2 3B shows great progress over Gemma 2B IT but still trails behind Phi-3.5-mini IT, which scores an outstanding 86.2 in GSM8K.
Long Context and Multilingual Abilities
- ARC Challenge (5-shot): Tests the model’s capability in reasoning. Llama 3.2 3B does exceptionally well, with a score of 78.6, slightly better than Gemma 2B IT but trailing behind Phi-3.5-mini IT at 87.4.
- NIH/Multi-needle: Here, Llama 3.2 3B shines with an 84.7 score, which is significantly higher than both Gemma 2B IT and Phi-3.5-mini IT.
2. Vision Instruction-Tuned Benchmarks

The second table focuses on vision-related benchmarks, specifically image and text-based tasks.
Image Processing and Understanding Benchmarks
- MathVista: This benchmark assesses visual mathematical reasoning. Llama 3.2 90B achieves a strong score of 57.3, better than Claude 3 — Haiku but just behind GPT-4o-mini.
- ChartQA and AI2 Diagram: These benchmarks assess how well the models understand and generate descriptions for charts and diagrams. Llama 3.2 90B performs impressively, scoring 85.5 in ChartQA and 92.3 in AI2 Diagram, showcasing its robust visual comprehension capabilities.
- DocVQA: Another benchmark assessing the model’s ability to understand documents with visual information. Llama 3.2 90B scores a commendable 90.1, slightly edging out Claude 3 — Haiku.
Text-Based Benchmarks
- MMLU (0-shot, CoT): In the reasoning-heavy MMLU test, Llama 3.2 90B scores 86.0, slightly outperforming Claude 3 — Haiku and close to GPT-4o-mini.
- MATH (0-shot, CoT): Llama 3.2 90B excels in this task, scoring 51.9, a significant improvement over Claude 3 — Haiku, but still behind GPT-4o-mini at 70.2.
- GPQA (0-shot, CoT): In the general purpose question answering benchmark, Llama 3.2 90B scores 46.7, better than Claude 3 — Haiku, but behind GPT-4o-mini.
- MGSM: This is a multilingual benchmark, where Llama 3.2 90B scores 86.9, a solid result, but GPT-4o-mini performs even better at 87.0.
Summary
- Llama 3.2 performs exceptionally well in a variety of tasks, particularly in tool use, reasoning, and visual understanding, showcasing a clear advantage over competitors like Gemma 2B IT and even Claude 3 — Haiku in several categories.
- Phi-3.5-mini IT generally leads in mathematics and reasoning, especially in the ARC Challenge and GSM8K benchmarks.
- When it comes to vision tasks, Llama 3.2 90B outperforms Claude in almost all the vision benchmarks, showing that it’s very capable in multi-modal tasks.
- GPT-4o-mini maintains leadership in specific text-related benchmarks, particularly in MATH and MMLU, but Llama 3.2 holds its ground well.
How Llama 3.2 Leverages Pruning and Distillation for Lightweight Performance
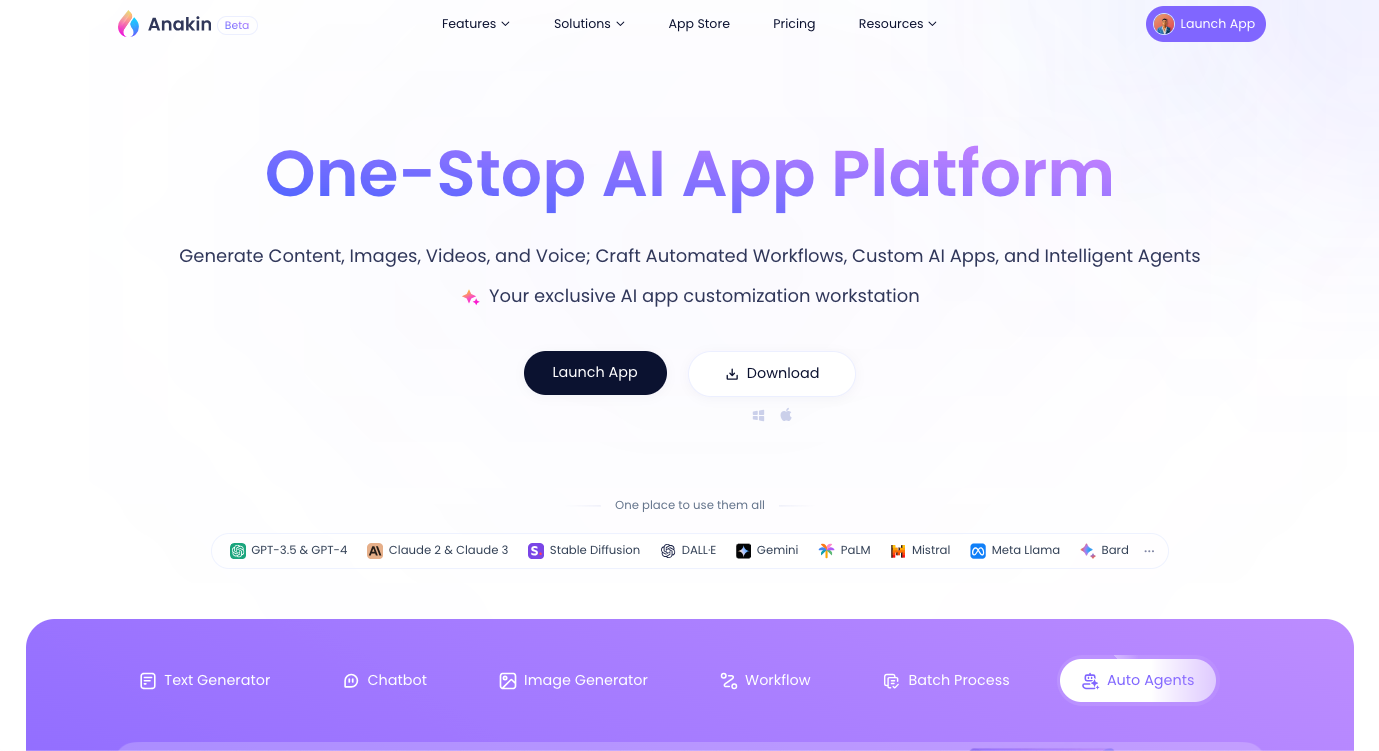
One of the standout innovations in Llama 3.2 is its ability to scale down without compromising on performance. Pruning and distillation methods were used to create the 1B and 3B models, which retain much of the power of their larger counterparts while being able to run efficiently on smaller devices.
- Pruning: By systematically removing non-essential parts of the model network, Meta reduced the size of the 1B and 3B models. The models maintain their performance due to intelligent weight and gradient adjustments.
- Distillation: Larger models like Llama 3.1 70B were used as "teachers" to guide the training of the smaller models, ensuring they retained high performance even after pruning. If you're curious about how this process works, you can explore a detailed breakdown in our AI Model Development blog.
Vision Capabilities: A New Frontier for Llama
Llama 3.2 introduces image reasoning for the first time in the Llama series, thanks to a new architecture that integrates pre-trained image encoders with language models. By aligning image and text representations, these models offer rich vision-language capabilities that outperform many closed multimodal models.
- Training with Image Adapters: Meta trained a set of adapters to connect image encoders with pre-trained language models. This process ensured that the vision models could retain strong text-based reasoning while also understanding visual inputs. For those looking to harness these capabilities for creative applications, the Deepfake AI Image Generator is a great example of how Llama 3.2’s vision capabilities can be applied.
Use Cases and Applications
Llama 3.2 is optimized for a wide variety of tasks, especially those requiring edge AI deployment and real-time inference. Some specific use cases include:
- On-Device AI: The lightweight models are perfect for mobile applications where privacy is paramount, as all data processing can be done locally. Apps like the Goodnight Message Generator benefit from Llama 3.2's fast, privacy-focused processing.
- Document-Level Understanding: The vision models excel at document analysis, making them useful in enterprise settings for tasks like financial document reviews and medical imaging. Similarly, our AI Floor Plan Generator offers a glimpse into how vision models can assist in structured image-to-text generation.
- Real-Time Visual Grounding: Applications that require understanding of images in real-time—such as drones, autonomous vehicles, or AR/VR systems—can benefit from the 11B and 90B models. These applications align with the work we've done in the AI Image Processing Tools on our platform, where real-time image understanding is critical.
Conclusion
Meta’s Llama 3.2 is a top performer in its class, leading the way for edge AI, vision tasks, and on-device solutions. The lightweight models demonstrate impressive performance, particularly for on-device use cases, making them ideal for privacy-conscious applications. If you're interested in exploring how these models can power your AI applications, check out our apps like AI Nude Generator or explore our full range of tools on Anakin AI.